The AI effect: high-performing Sentinel-2 cloud mask goes global
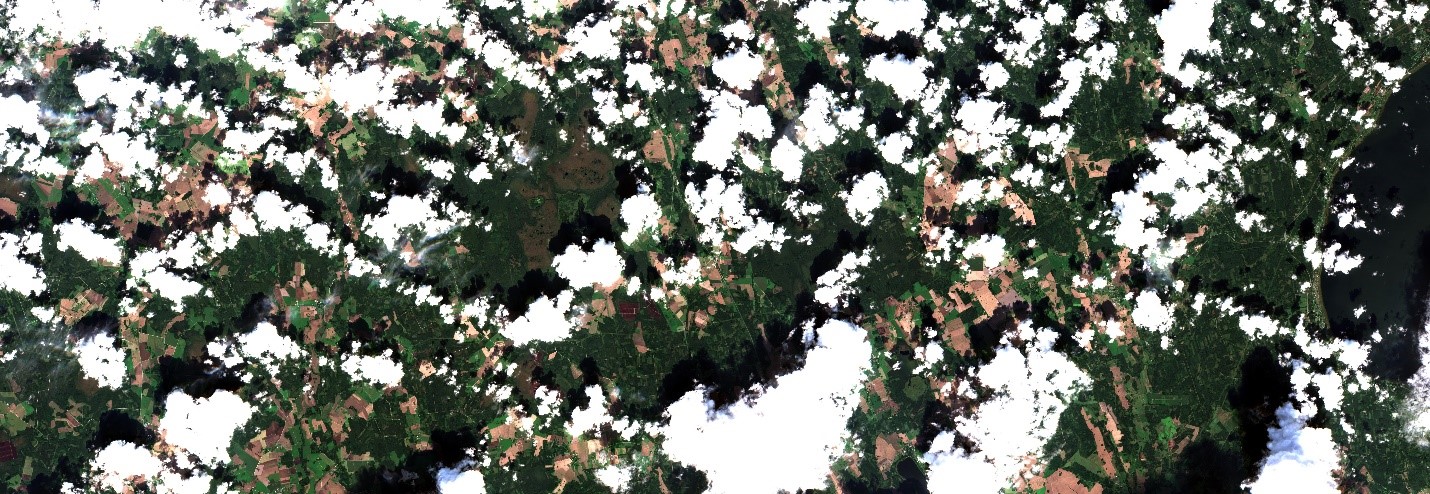
After releasing its free and open-source cloud mask for Copernicus Sentinel-2 data, Estonian company KappaZeta is now working on enlarging the model from the Northern European summer season to year-round global coverage. Developed in conjunction with ESA Φ-lab, KappaMask is already outperforming similar approaches and uses Artificial Intelligence (AI) and active learning techniques to optimise automatic data labelling.
Although well established as a gold-standard provider of Earth observation (EO) insight, the data from Sentinel-2, like all optical satellite imagery, needs to have cloud and cloud-shadow areas identified and filtered out. Creating a cloud mask, effectively a stencil that removes unwanted data, is an essential step for virtually any EO application, and as ESA has shown with the Φ-sat-1 experiment, masking can be done effectively at source through onboard processing on the satellite. For Sentinel-2 users however, free, accurate and user-friendly cloud masks are currently few and far between. While masking is relatively simple when studying small areas, large stacks of imagery require automated pre-processing in order to provide timely, valid data to the user.
KappaZeta set out to provide an AI-powered, free-of-charge solution for Sentinel-2 data users worldwide. In an initiative funded by ESA Φ-lab, development commenced in 2020, with Phase 1 focused on Northern European summer-season conditions. Refining the mask was aided by the adoption of active learning, an approach which selects the highest impact samples for labelling. “We needed a reference dataset to train and test our model,” explains KappaZeta CEO Kaupo Voormansik. “Manual labelling of satellite imagery is a slow and expensive process, but as interest has grown in Deep Learning, the active learning methodology has proven to be a powerful tool for efficiently creating high-variety reference cloud masks using limited resources.”
Phase 1 was completed in August 2021, with the outcome published in a research paper and the initial version of KappaMask released to the public. The European model proved to be highly accurate and in fact performed better than comparable products, with particularly noteworthy results in the detection of cloud shadows and small fragmented clouds – a problematic area for some previous cloud masks.
The successful release of the Northern European summertime mask was followed by Phase 2, which aims to extend the model to the rest of the world over all seasons. This entails both improving the Phase-1 model architecture and obtaining a global reference dataset. For the latter, KappaZeta has used a combination of existing labelled datasets and its own labelling, the plan being to add 5000 newly segmented sub-tiles (each consisting of a 512 by 512 pixel area) to improve model accuracy. With an eye once again on efficient working, the team has picked the sub-tile locations based on Sentinel-2 data download statistics, thereby selecting according to user interest rather than aiming for a blanket global coverage.
Nicolas Longépé, Φ-lab data scientist and one of the ESA supervisors for KappaMask, recognises the effectiveness of the company’s research paradigm: “KappaZeta has used a smart approach for developing its cloud mask, with active learning and demand-based coverage helping to achieve the right trade-offs in terms of precision versus effort. Indeed the Phase-1 results have already shown KappaMask to be one of the most accurate free-to-use cloud masks, and once complete we fully expect the product to significantly enrich the analysis toolbox of Sentinel-2 data users.”
“We are also happy to support the project to see how KappaMask compares with other available solutions,” added Valentina Boccia of the ESA EO Ground Segment Department. “KappaZeta’s work convincingly illustrates how innovative AI techniques could be integrated into the mainstay of Sentinel-2 data processing.”
KappaMask is scheduled for release as a cloud-masking web service later this year. The reference dataset and source code will be freely available, and details of the model and the accuracy validation will be published in a forthcoming paper.
To know more: Sentinel-2, KappaZeta, Φ-lab Explore Office
Share