Case study: Computer vision & food security issues
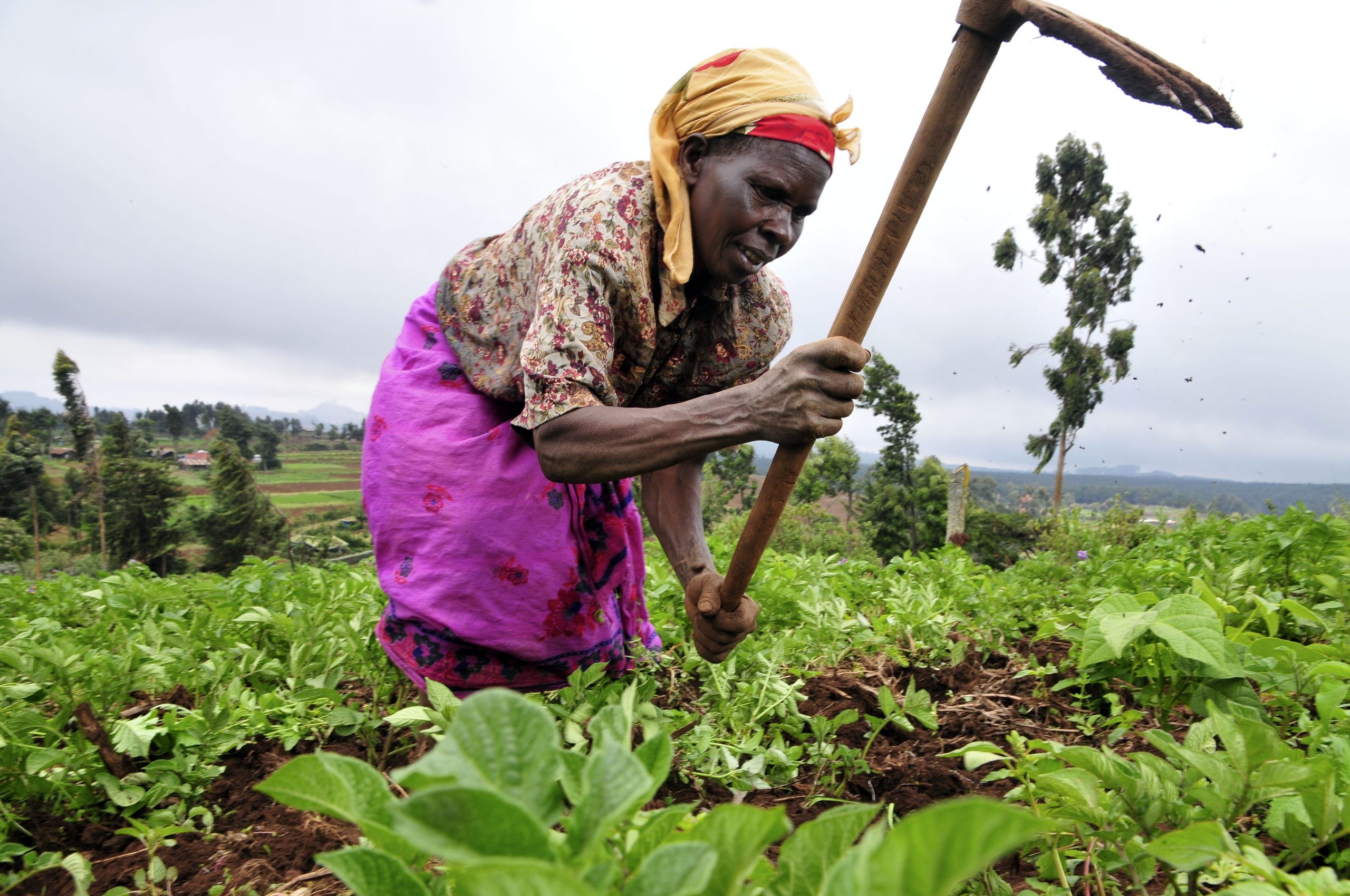
How images of cats and dogs can help solving food security issues?
Current development in Artificial intelligence shows the strong ability of computers to learn information about our world. One of the most applicable methods artificial intelligence can offer is generalization of information based on some examples. These approaches applied to a certain problems resulted in a great success. For example, in computer vision domain, machines are now capable to classify any image into one of around a thousand of object categories with the accuracy comparable or even better than humans. The roots of the successful methods come from biomedical discoveries of how mammal brains analyse visual information.
This way is now being reflected in artificial intelligence by so-called deep learning. But our brain is capable to analyse not only images from our daily life environment, but also can recognize objects in satellite or drone images. So, why do not apply deep
learning techniques for Earth observation images? The only problem is that to achieve a good results, deep learning methods need a huge number of learning examples, which is typically not assured in Earth observations.
This is why Φ-lab is conducting a research on how methods and knowledge developed for computer vision can be transferred to Earth observation images via a process called transfer learning. Our first success was to show that general information learned by deep neural networks pre-trained on images of objects like cats and dogs can be very useful in crop types mapping on drone images.
This achievement is now being explored with our partners, World Food Programme and UNICEF Innovation for improving agricultural monitoring which helps manage food supplies and children’s welfare in developing countries.
Rohingya children playing at a UNICEF child friendly space. Credit : Anna Dubuis /DFIDComputer vision & Food security issues
Post contributed by Artur Nowakowski.
Share